Basic MLB DFS Strategy: Tracking the Statistics That Matter
It can be easy to get bogged down in statistics when researching daily fantasy baseball options - so we’re here to help simplify things for you.
The key to coming up with a solid research routine is being able to establish which statistics are most relevant to daily fantasy. In truth, not all of them are; some represent too small a sample size to be reliable, while others offer little or no predictive value whatsoever.
So where do you begin?
For starters, it’s difficult to identify anything as a statistical trend until x number of games have been played by each team. The “x” in the equation is up for debate, but generally you need at least a month before being able to make declarative statements about any trends.
Next, you’ll need to be able to shut out the noise and focus on the statistics that matter most. Many beginner players make the mistake of focusing too heavily on past performances; while they offer limited predictive value in certain cases, DFS success comes from being able to project. It’s about looking into the future - not gazing into the past.
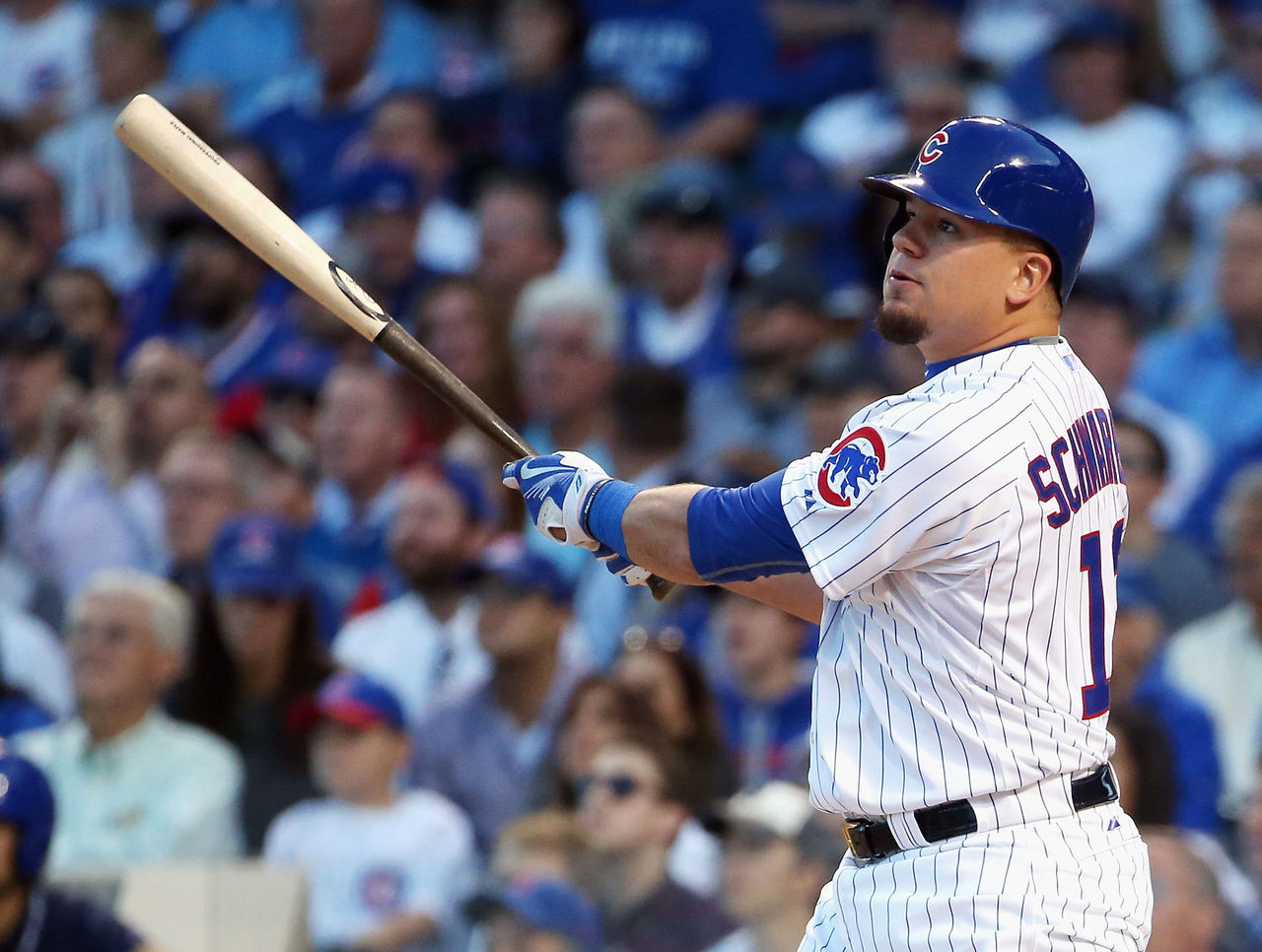
Consider the example of a player who puts together a week in which he hits .550 with four home runs and 11 RBIs. By the end of the week, that player’s salary will have climbed considerably. Do you select that player, higher salary and all, because he’s on a hot streak? Or do you look elsewhere, anticipating the difficulty that player will have maintaining his torrid pace?
When laid out this way, the answer appears obvious: do the latter. But recency bias in statistics has a powerful hold on people, skewing their view of players who are either underachieving or overachieving - and those poor judgments can make a significant difference in daily fantasy.
Here’s a breakdown of the most common stats casual players access when conducting DFS research, and whether those stats are helpful or harmful:
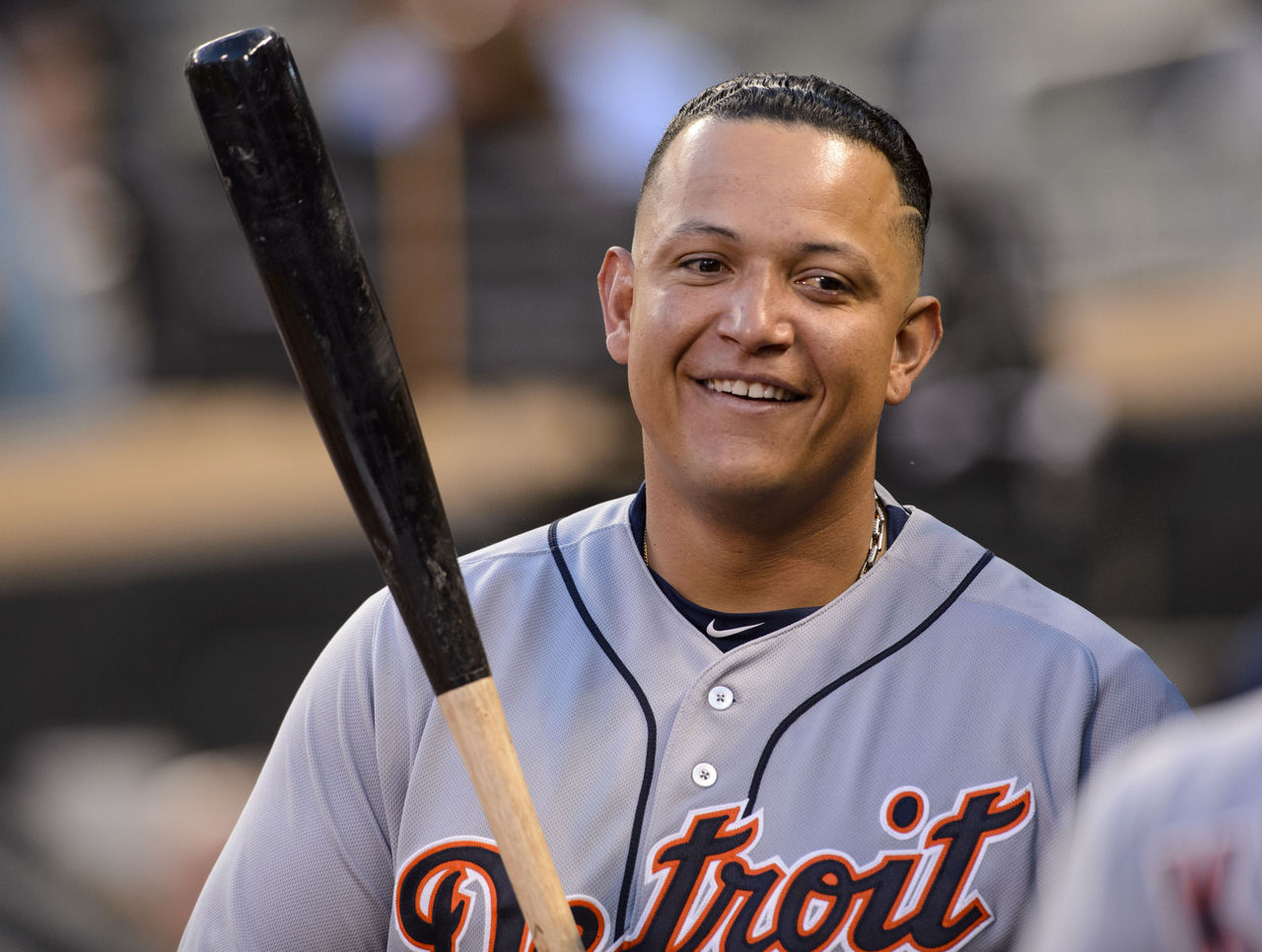
Core Stats (AVG, HR, RBI, R, SB, W, K, BB, ERA): These are useful as base measurements of a player’s daily fantasy value, but that’s all they should represent - particularly early in the season, when such statistics are often skewed due to small sample size.
Home/road splits: These are more useful for pitchers than for hitters - and generally follow a predictable pattern in which the vast majority of pitchers are stronger at home than on the road. Don’t spend a lot of time looking for advantages here, particularly when it comes to hitters.
Batter/pitcher matchups: These are virtually worthless in DFS; pitchers and batters have faced off so few times that there’s no measurable benefit to using the matchup stats.
Lefty/righty splits: DFS users may find significant value here. Pitchers tend to fare better against like-handed hitters, so knowing just how dominant a pitcher is against those batters - and conversely, which hitters are strong against like- and opposite-handed hurlers - is vital.
Last 10 games/starts: Again, we’re dealing with such a small sample size that there’s little value here. There’s a real danger in riding a player’s hot streak - and the boosted salary that goes with it - or ignoring a player in a slump even though he may represent great value.
There are dozens of “next-level statistics” that more hard-core DFS players might use, but we’re going to focus on four that carry more weight than most:
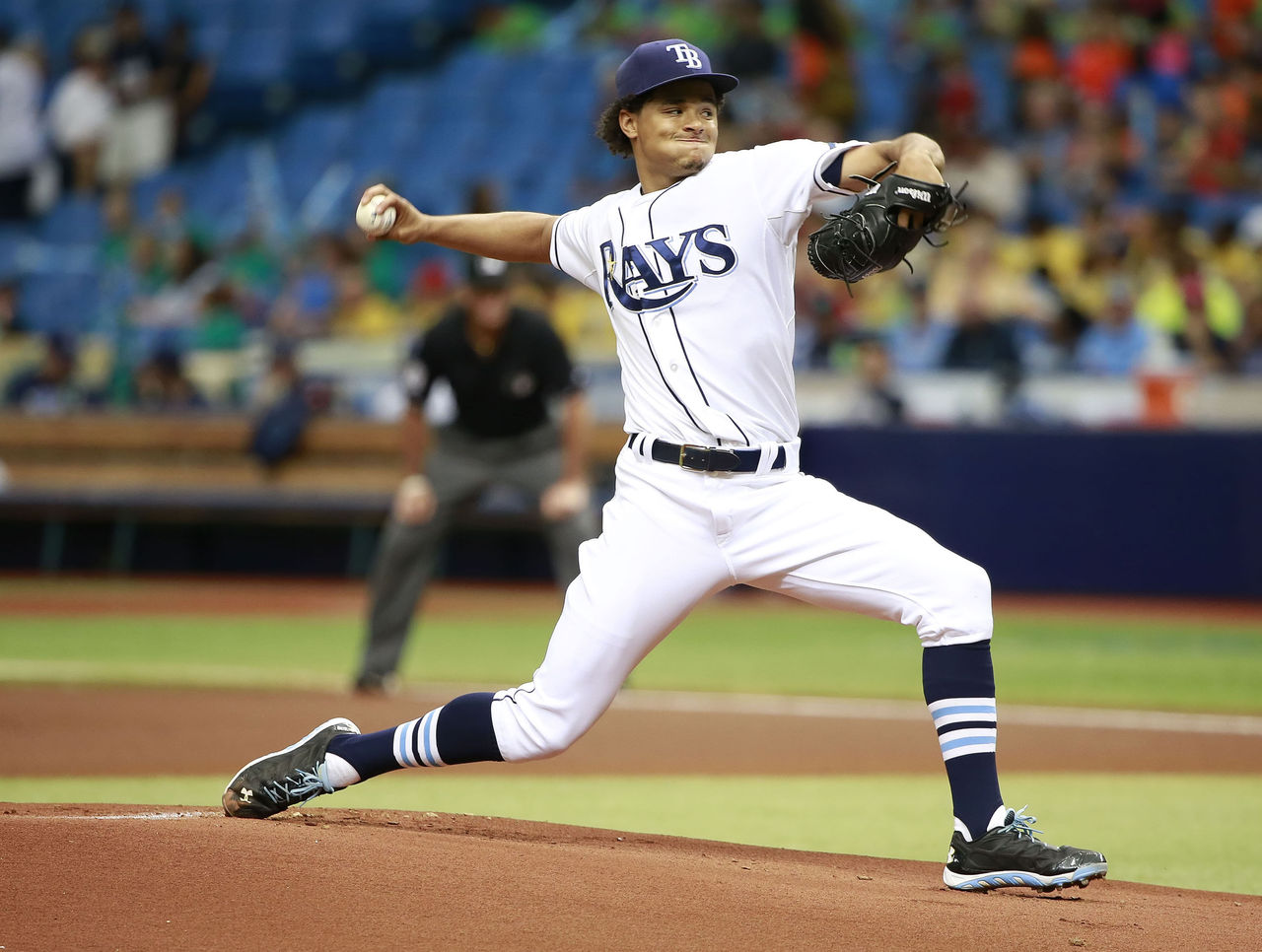
Strikeout Rate: This stat comes in two parts - individual pitcher strikeout rate and opposing team strikeout rate. Both are incredibly useful in determining which pitchers represent the best value on a given night. The higher the K rate on either side, the better the choice.
Weighted On-base Average (wOBA): This is used to measure true offensive value of a hitter or team via a formula that considers all possible at-bat results and provides different weights for each. The average player will record a wOBA of roughly .320, so use that as a baseline.
Batting Average on Balls in Play (BABIP): This measures how often a player reaches base when he hits a fair ball. A high BABIP - say, .400 or higher - suggests that a hitter has been lucky (the league average is around .300), making him a prime regression candidate.
Expected Field Independent Pitching (xFIP): This stat measures a pitcher’s ERA assuming standard fielding and BABIP rates. If a pitcher’s xFIP is lower than his ERA, that means he has been victimized by poor fielding or a high BABIP that will likely improve over time.
Mastering the four statistics above won’t necessarily translate directly to victory, but it will give you the tools needed to succeed on a regular basis.